DATATRON
ROLE
Product Designer
Duration
July – August 2019 (2mo)
TEAM
Data Scientist
SWE.Backend
SWE.Frontend
Tools
Sketch
Responsibilities
Product Design (Concepting, Prototyping)
Background
Over the years, increasing traffic congestion in the Bay Area has prompted a shift towards more public transit and commute options. Various options such as BART, CalTrain, VTA, and Uber/Lyft are available, but individuals frequently spend more time and money by relying solely on a single commute option.
Ford Motor Company approached us with a request to help them bring machine learning to a new transportation service they were launching in the coming months. With a timeline of 1 month for design, development, and testing, I worked with our team to build a machine learning-powered dynamic pricing tool.
With their interest in breaking into the rideshare market, Ford came to Datatron with the question
With their interest in breaking into the rideshare market, Ford came to Datatron with the question:
Problem statement
Goal
Process
With multiple directions to take, I worked with our data scientist and engineering team to research into the space and the features requested.
Research
Analyze Bay Area transit services
Understand how each Bay Area transportation and commute services operates in relations to factors that affect pricing and ridership.
Ideation
Breaking down the model
Work alongside our team to create a configurable UI that outputs dynamic pricing models from ridership parameters.
Research
Ideation
Analyze Bay Area transit services
The project had two phases: developing a machine learning model to determine ridership and designing an interface to consume and visualize the data provided by the model.
In a report, our data scientist Pietro summarized the effects of factors like seasonality, time of day, and geographic distribution on transportation services. The report provided the knowledge I needed to proceed with the UX of the tool.
Caltrain ridership is affected by the time of day and the direction a rider is going during peak hours during a typical work week.
Research
Ideation
Breaking down the model
The interface was designed to allow users to configure a ride-sharing options that would provide an output model that Ford could use with their own service.
I worked with Pietro to identify the best approach to break down the model parameters as well as the data visualizations that plot the changes in ridership and pricing.
Next project
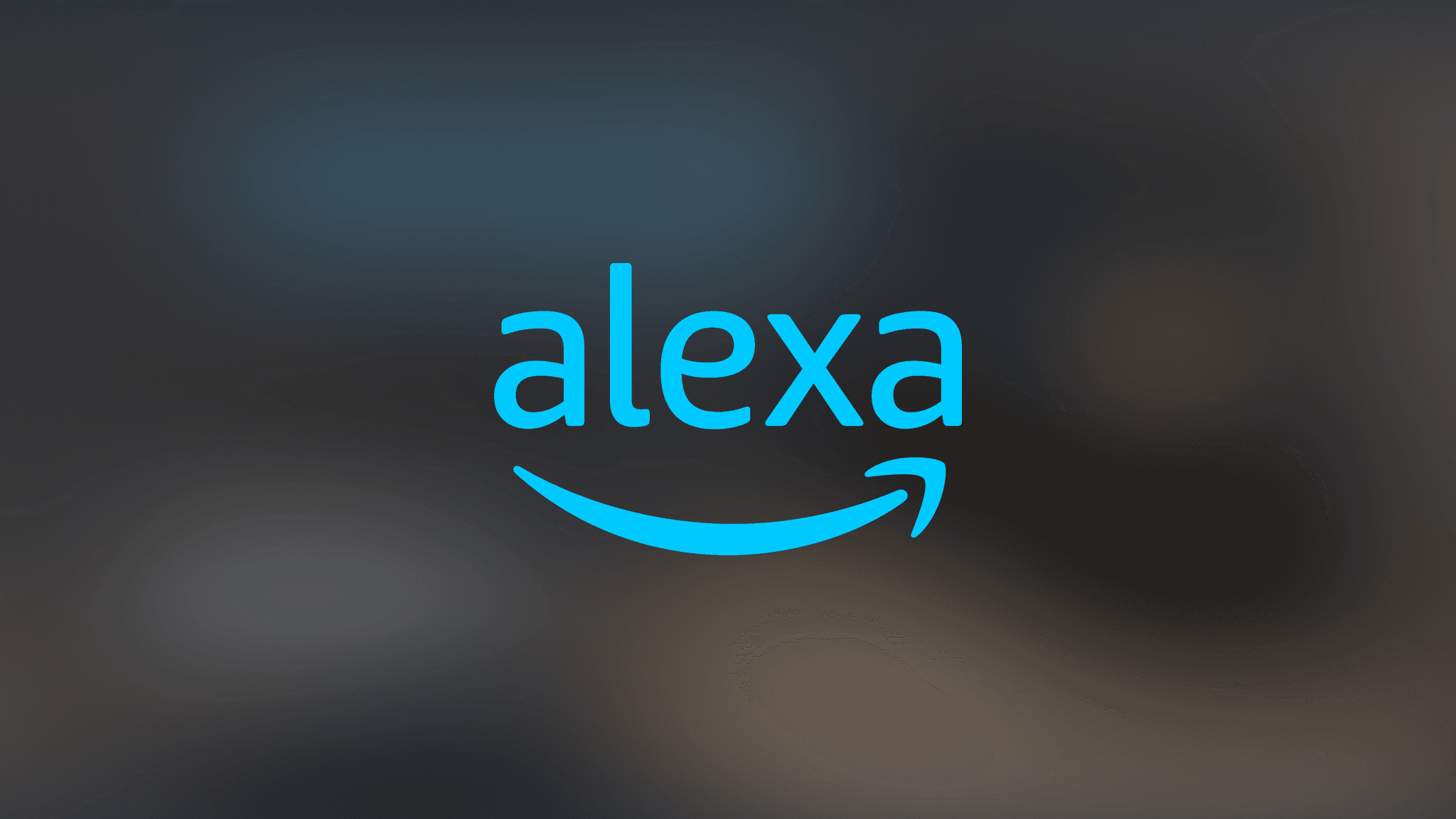
Alexa Smart Proprties
Product Design